by Snigdha Joshi
6 minutes
Artificial Intelligence and Machine Learning Transforming Drug Discovery
How AI and ML are reshaping the drug discovery process by improving speed, accuracy, and efficiency.
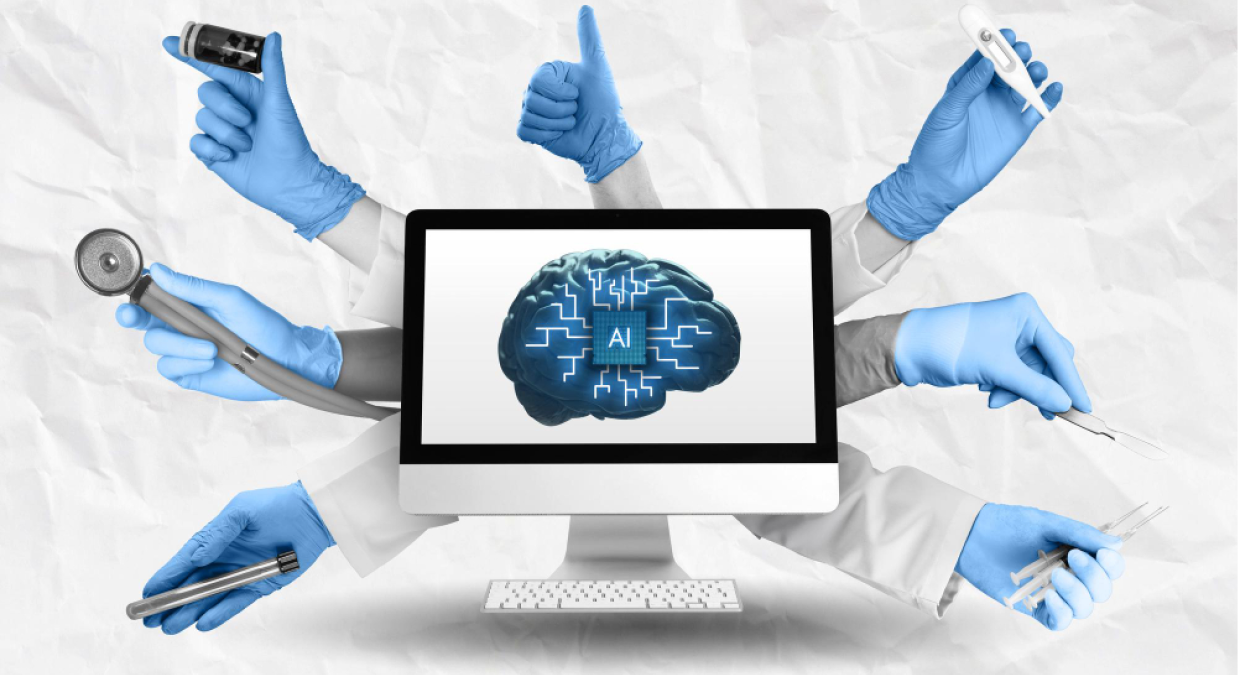
Developing new drugs and pharmaceutical products is a complex, resource-intensive, and expensive process with an unfathomably high failure rate. To reduce the overall burden and meet the high requirements of drug discovery, pharmaceutical companies are looking to integrate artificial intelligence (AI) and machine learning (ML) into their drug discovery processes. AI-powered systems have already been incorporated into various operational processes in pharmaceutical companies. For example, GenAI and AI-based chatbots are used regularly.
The integration of AI and ML in drug discovery is promising. AI and ML-powered solutions have improved the efficiencies and success rates of various processes in several industries. This potential can also be translated into drug discovery. However, as drug discovery is a complex process, AI and ML solutions can be integrated into several key steps to speed up the timeline.
What’s the scope?
AI and ML can transform drug discovery by contributing to some key areas in the drug discovery pipeline:
Target identification and validation
Target identification is time-consuming because researchers must analyze thousands of proteins and molecules from patient samples to identify those that play key roles in disease transmission and progression. AI and ML models can analyze large datasets to identify new drug targets, reducing the time required. Furthermore, ML models can predict disease-associated genes and proteins in patients, which need only to be verified, speeding up the drug development timeline.
Compound candidate screening
Candidate screening involves testing compounds against the identified target to determine their potential for treatment. However, there are thousands of libraries to screen from, making this a time and resource-intensive process. However, AI models can screen the available libraries and list prospective compounds.
Predicting drug-drug interactions and side effects
AI and ML models trained on molecular interaction data can predict the adverse effects of drug candidates when used with other drugs. The drug candidate can then be modified to reduce such interactions or side effects. Furthermore, these models can also predict the drug candidate's short- and long-term toxicity, preventing possible disruptions later in the development phase.
Personalized Medicine and Precision Drug Development
As personalized medicine and precision drug development increase interest, AI and ML models can accelerate their integration. AI models can personalize the drug according to the patient’s genetics, biomarkers, and lifestyle. ML models can predict responses to personalized medicine.
Automating processes
AI can also automate laboratory processes involved in drug development. For example, AI models can speed up high-throughput screening and drug testing. ML models can analyze lab results and optimize existing experimental processes to meet the benchmark.
AI and ML are no longer a far-off dream—they’re a reality implemented by many pharmaceutical companies.
Who’s using AI and ML?
AlphaFold
Google Deepmind’s AlphaFold has predicted more than 200+ million protein structures. The AlphaFold Server can predict how proteins interact with various biomolecules. AlphaFold 3, the most recent model reported by Google Deepmind, can accurately predict the structure of proteins, ligands, DNA, RNA, and biomolecules and their interactions with other materials.
BenevolentAI
BenevolentAI, or Benevolent Platform, is among the most studied AI-driven drug discovery and development tools. The platform uses AI to solve specific drug discovery problems. It can be used for biomarker assessment and drug repurposing. The technology can be integrated into a company’s platform using Benevolent’s API offerings.
Insilico Medicine
Insilico Medicine offers a drug discovery software suite named Pharma.AI to speed up the drug discovery process. PandaOmics enables target discovery. Chemistry42 is an ML-based platform that can be used to find novel lead-like molecules. inClinico can be used to design and predict the success rate of clinical trials.
Atomwise
Atomwise’s AtomNet uses a deep convolutional neural network for drug discovery. AtomNet facilitates structure-based drug discovery and is currently tackling 600+ unique disease targets. The platform has been actively used for drug research for more than 2 years.
NVIDIA Clara Discovery
NVIDIA’s Clara Discovery is a suite of frameworks, applications, and AI-powered models that enable computational drug discovery. It supports genomics workflows, CryoEM pipelines, virtual screening, molecular simulation, and protein structure prediction.
These technologies are being used to hasten drug discovery to meet the high global demand for more efficient and effective medicines. However, integrating AI and ML-powered solutions is still nascent, with few pharma companies integrating them in regular operations. This is primarily because of three significant challenges.
Challenges for AI and ML
Data quality and availability
AI and ML models are trained on high-quality data and require high-quality structured data to provide reliable, accurate, and reproducible results. In reality, most available data is poor-quality, unstructured, and fragmented (from various sources), requiring data pre-processing. Furthermore, while obtaining and using this data, pharma companies must comply with local and international data privacy and security guidelines. Data availability, privacy, and security challenges must be addressed before AL and ML models can be successfully and widely integrated.
Regulatory hurdles
AI and ML models lack regulatory support because they are not transparent with results—the model may recommend Method A over Method B, but won’t explain the underlying reason for this recommendation. This creates scepticism among stakeholders, including regulatory bodies, who raise concerns regarding the model’s ethical framework. Hence, regulatory guidelines on using AI and ML models are still vague. Regulatory support (or opposition) for using AI in drug discovery has not been established, making implementing existing models tricky.
Financial hurdles
While AI can save time and decrease costs during drug development, implementing AI and ML models is expensive. Companies must hire specialized staff with technical expertise to develop and train models. Often, this may be just as expensive. Furthermore, after implementation, companies need to hire additional staff to pre-process all available data so that it can be used by the model, which increases the daily operational cost of the model.
Will we overcome these challenges?
We’re optimistic that the pharma industry will overcome these hurdles and that AI and ML models will be implemented. While these challenges seem pretty significant in 2025, they will not seem so in hindsight. Suppose the recent reports on DeepSeek AI’s lower development costs than ChatGPT are to be believed. In that case, the development of AI and ML models will become cheaper, resulting in widespread implementation and a boom in drug discovery.
FAQs
1. What are the benefits of machine learning in drug development?
Machine learning improves efficiency, reduces costs, and increases the accuracy of predictions in drug R&D.
2. Can AI predict drug side effects and interactions?
Yes, AI models can forecast potential side effects and interactions, helping avoid failures in later stages.
3. Which companies use AI for drug discovery?
Leaders include DeepMind (AlphaFold), BenevolentAI, Insilico Medicine, Atomwise, and NVIDIA.